Artificial Intelligence (AI) technology is reshaping the way enterprises extract insights from data. The Gartner’s most recent hype cycle report on Types of AI points out that AI is the most favourable CIO technology initiative for the next five years as a source of business transformation.
PwC predicts that by 2030, AI can potentially contribute around $15.7 trillion to the global economy.
Many organizations believe that AI is not just a business enabler, but that it is having fundamental impacts on the function itself. AI is automating some long-standing functions to deliver upon their demand for innovative approaches and greater involvement from the IT departments.
Long story short, AI is a big deal. Various flavors of cognitive capabilities make AI a success. It becomes critical for enterprises and business leaders to understand the types of AI and the impact that it will have on the IT operations and market.
Five types of Artificial Intelligence
1. Machine Learning
In the current scenario, machine learning (ML) is the most relevant and popular subset of AI. The Executive’s Guide to the real-world AI, a recent report by Harvard Business Review Analytic Services, stated that ML has been around for years and has matured into a technology.
ML allows computing devices to self-learn from data and implement those findings without any human intervention. Often when a solution/result is hidden in a huge data set, ML is really very helpful. ML is outstanding at data processing and pattern recognition as it takes a fraction of the time that a manual process would take.
Use Cases
Just to list a few of the ML use cases in the real world, ML is used in fraud detection, portfolio management, and risk analysis for financial services. It is also used for targeted marketing campaigns, GPS-based fleet tracking solutions and travel predictions.
2. Deep Learning
Any computer program that does something smart is powered by AI, which is an umbrella term that holds ML as a subset. Here, Deep learning is a subset of ML that works towards mimicking the human mind really closely.
CompTIA explains that deep learning allows computer programs to process the problems in multiple layers, simulating the human brain analytical capabilities. The deep learning technologies extract the meaning out of information to build context. While, to increase the chances of getting the correct conclusion, deep learning empowers computer applications to understand the various components of the inputs such as text or visual images.
One explanation by deep AI says that this technology learns from processing the labelled data that is provided during the training. To process the labelled data, deep learning uses neural networks (yeh, that’s what they call them). The output of this processing is used to learn the attributes of the input that were required to come to the correct output. As soon as the sufficient number of inputs have been processed as examples, the so-called neural network can start processing new data.
Use cases of Deep Learning
The content and product recommendations we get on Netflix and Amazon are powered by deep learning. Moreover, Google’s voice and image recognition algorithms have deep learning inside. The deep learning’s potential to analyze a huge amount of multi-dimensional data makes it an ideal pick for preventive maintenance systems. McKinsey found in its studies that neural networks improve AI’s capabilities in terms of improving the product form data such as audio and image which is ingested by sensors, including some inexpensive sensors. Manufacturing units can utilize AI capabilities to predict equipment failures which helps in reducing downtime and operational costs.
3. Natural Language Processing
When AI focuses on human linguistics, it is called Natural Language Processing (NLP). The most basic possible explanation of NLP is that it makes it possible for humans to talk to machines. In other words, it enables computers to comprehend and manipulate human languages.
So basically, NLP is understanding the human language and sewing something based on the understanding. There are a number of subsets of NLP which includes Natural Language Understanding (NLU) and Natural Language Generation (NLG). NLU refers to reading comprehension of machines while NLG refers to the transformation of the data into human language. To effectively comprehend and process unstructured text and speech datasets, NLP might require to utilize both machine learning and deep learning methodologies.
Use cases of NLP
NLP empowers computer programmes to identify keywords and intents of languages, process or translate it and generate a response. Chatbots, emails, social media feeds, or some very complex documents, wherever the human language is involved, there is a chance to deploy NLP capabilities.
4. Computer Vision
Computer vision empowers computers to see the world the way that humans do. It literally equips the capability in computer programs to interpret and understand the visual world. Computer vision includes training the computer programs using deep learning models with digital images. It allows machines to identify and classify objects accurately.
While the existence of computer vision dates back to the 1950s, the advancement of computing technologies and hardware are setting off the renaissance of computer vision. A survey by Harvard Business Review Analytic Services stated that 82% of the survey respondents said that they would have adopted computer vision or would access and implement computer vision within two years.
The deep learning models empower computer vision to learn viewing and interpreting the world in a way similar to humans. It also allows computer vision to get better at pattern detection in images and other visual data sets. The continuous innovation in this type of AI technology in the recent decade has improved accuracy rate from 50 percent to 99 percent. The modern computer vision-enabled systems can detect and react to visual inputs very quickly and are more accurate than humans in some cases.
Use cases
The computer vision technology is empowering driverless automobile solutions such as Tesla’s Autopilot and Google’s Waymo. To be noted that AWS (Amazon Web Services) has invented a programmable deep learning-enabled camera system which can be used by enterprises to create their own applications.
5. Explainable AI (XAI)
This type of AI works on improving the reliance. We as humans, need rational justification and transparency in order to trust a decision. On the contrary, AI technology is like a black box: input, then output – with little or no transparency to how it got to the conclusion. Many IT leaders say that understanding the decision-making process is mission-critical in order to rely on it. As the reliance on technology is increasing day by day, the need for understanding the ‘thought process’ of AI will be crucial to improve it over the time.
Use cases
Many organizations across the world are developing ML techniques that allow humans to understand and effectively manage AI technology. Defence Advanced Research Projects Agency (DARPA), an agency responsible for the development of emerging defence technologies for the United States Department of Defence, believes that the XAI capabilities will result in third-wave AI systems. The need for XAI will increase in the healthcare, insurance, manufacturing, and automotive industries.
AI is Reshaping IT
AI technologies have started changing the world of IT. Here are the number of ways IT leaders across the world think the Types of AI will transform the IT industry.
1. IT is the biggest AI Consumer
For a long time now, the IT world has been using tools that automate traditional break-fixes and IT service desk processes. In the areas of service desks, AI is bringing cognitive computing and improved automation to leverage repetition of customer service operations.
This is not the only area where AI-enabled hyper-automation is improving the IT functions. IT has gone beyond partnering with Types of AI, it actually has become the major consumer of AI. IT has started leveraging AI capabilities for system and security management.
2. Shadow IT Might Expand
AI capabilities are helping IT services proliferate outside the tech core. Functions across the enterprises in various industries have started utilizing machine learning models i.e. robotic process automation (RPA) adoption and self-service data science and analytics tools. Of course, the line between ‘shadow IT’ and ‘self-service’ depends on the company culture itself.
3. Data Science requires IT collaboration
AI and automation is fueling the effectiveness and efficiency of many mainstream enterprise applications such as CRM and ERPs. But the AI will require a greater partnership with IT to come up with more advanced AI-powered enterprise solutions and tools.
Enterprises across the world are preparing to scale their use of data science and AI capabilities. They would need a deeper understanding of the IT capabilities to build AI-led solutions. In simple terms, advanced AI solutions will require an intense collaboration between IT engineers and data scientists. While both data science and IT are a broad area by themselves, enterprises would need to configure teams that can work together to build effective Types of AI solutions.
4. IT and Data Science need to share tools and techniques
The collaboration between data science and IT require each group to get familiar with each other’s tools and techniques. IT engineers will be required to read source codes that ingest data from local data sets, understand feature engineering and exploratory data analysis, and need to become fluent in associated techniques of statistics to generate insights. All this knowledge will help IT engineers to refactor and modularization the source codes, so that the code can be ope-rationalized in enterprise IT solutions.
Similarly, the data scientists will need to learn to pull data via database connectors or APIs. They will also be required to store and process the data sets in structured stores and write modular code for downstream consumption.
5. AI governance takes center stage
As the enterprises are implementing AI-powered automation and processes, the risk of regulatory compliances has increased. Gartner has pointed out the significance of policy creation to fight AI-related bias, discrimination, and many other issues.On the area where the IT and Data Science leaders join forces, Gartner suggests that enterprises put their focus on the following three points:
- Data algorithm transparency requirements
- Trust in data sources and AI outcomes
- Ensuring AI ethics and accuracy by diversifying the type of data, algorithms, and viewpoints.
While the real risk of AI governance does not associate with hypothetical future artificial superintelligence, the real concerns include issues such as algorithms driving internet search engines or personal assistant systems that already have more than adequate knowledge of every individual and their preferences. The massive amount of data we produce and provide voluntarily requires AI techniques to transform into insights. Types of AI is based on complex computer algorithms which can have errors.
For example, an autonomous vehicle can decide to run over a pedestrian to avoid vehicle collision. Another point of view is that the enterprises that utilize advanced AI solutions would require less human workforce, thus generating more unemployment. Such ethical dilemmas are increasing the need for AI regulation.
Some Final Thoughts
The Artificial intelligence technologies are realizing the automation of everything. We’ll be soon having all sorts of autonomous systems and operations. The combination of the Types of AI, data science, and sensor technology will make business operations more efficient and productive. In our daily lives, AI will be utilized to power the most frequent as well as creative tasks such as language translation and augmented creativity. Types of AI systems are already helping those who have limited artistic talents to produce original and creative works of art.
There are various theories and beliefs about the future of Artificial intelligence, but one thing is certain, it is coming and we’re already in the direction where AI will be a part of our lives. Companies across the globe are investing and experimenting with AI capabilities to figure out methods that will make the world a better place to live.
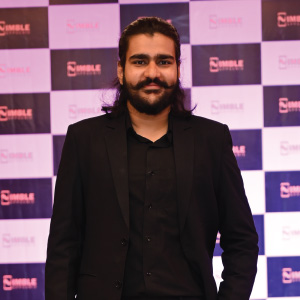
Udai Singh is a senior content writer with over 6 years of experience in creating content for FinTech, eWallet, EdTech, and App Development. He is an expert in simplifying complex concepts and creating engaging content that resonates with the audience.
Table of Contents
No Comments